OSaaS - Optimizing traffic Signals as a Service
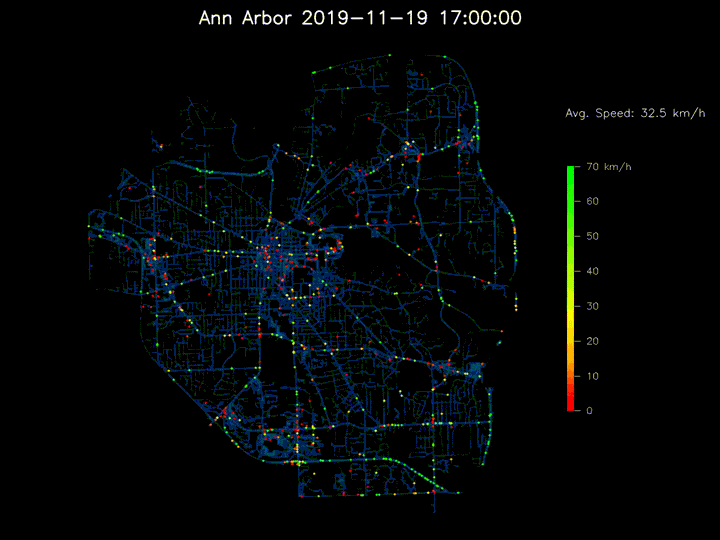
Related Publications
PDF Video DOI WSJ UofM News AP news Local 4+ Roads and Bridges
Patents and Technology Transfer
- Patent: Henry Liu, Xingmin Wang, Zachary Jerome, Shengyin Shen, and Zihao Wang. “Optimizing Traffic Signals Using Partially Observed Vehicle Trajectory Data”. US Provisional Patent Application 18/308,996. Filed on April 28, 2023.
- This technology was licensed to General Motors through the University of Michigan’s technology transfer program from 2023 to 2024. We are further developing OSaaS through the USDOT SMART Grants and moving towards commercialization via the NSF’s Innovation Corps (I-Corps) program.
Overview
OSaaS is a traffic signal control system that utilizes data from connected vehicles (CV) as the only input. The main advantage of CV data over traditional fixed-location detectors is its scalability – it can be collected from almost every intersection and roadway through various resources such as en-route navigation, roadside assistance, and ride-hailing. However, the low market penetration rate results in sparse and incomplete observation. To address this issue, I developed a new stochastic traffic flow model. Building on this model, I created an integrated traffic signal re-timing system called OSaaS (Optimizing traffic Signals as a Service). A real-world citywide test in Michigan demonstrated that the system decreased the delay and number of stops at signalized intersections by up to 30%. By eliminating the need for physical detectors, OSaaS significantly reduces the cost for traffic signal re-timing. Thanks to the scalability of CV data, it can be deployed at every intersection.
This research has been published in Nature Communications, making it one of the earliest transportation engineering articles featured in the Nature series. It has been covered by news outlets including University of Michigan News, the Associated Press, and the Wall Street Journal.
Probabilistic time-space (PTS) model
One major limitation of CV data is its sparsity, resulting from a low penetration rate (typically below 10%). To reconstruct the overall traffic state from these incomplete observations, a traffic flow model is usually needed. However, most existing models are not well-suited for low penetration rate CV data, as they are typically designed for fixed-location detectors or fully connected vehicle environments.
In my recent publication, I introduced a new stochastic traffic flow model called the probabilistic time-space (PTS) model, which uses a simple point-queue process to describe traffic flow dynamics near signalized intersections. While modern research often gravitates towards increasingly complex methods, the strength of this model lies in its simplicity, achieved through strategic reductions. One key insight is to disregard heterogeneous driving behaviors and assume that all vehicles only have two states: stop or go. This assumption is valid for low-penetration-rate trajectory data, where most of the uncertainty stems from incomplete observations and the stochastic nature of the arrival process.
Another innovation is the change of variables: by replacing time with free-flow arrival time, the free-flow travel time is eliminated, making overall vehicle travel time dependent solely on queueing time. I refer to this new coordinate system as Newellian coordinates, which also significantly simplifies the mapping between the point-queue representation and vehicle trajectories. By projecting the point-queue representation back into spatial-temporal space, we create the probabilistic time-space (PTS) diagram, which quantifies and visualizes the distribution of vehicle trajectories in the time-space diagram (see figure above).
The PTS model has a perfect match with near-intersection CV data, which directly provides vehicle stop location and duration. This is another main reason that inspires me to develop a queueing-based model. Based on the PTS model, I also developed different methods to estimate the overall traffic state with low penetration rate CV data. For example, in my most recent preprint, I introduced a Bayesian estimation method, which provides distributional estimation results for unknown traffic states and parameters.
Field Implementation
The PTS model and associated estimation method enabled us to establish a “digital twin” of real-world traffic. It could be used to predict what would happen if the signal timing was changed. I thus further developed optimization programs to optimize the signal timing parameters to reduce the delay and number of stops. This leads to a traffic signal re-timing system called OSaaS (Optimizing traffic Signals as a Service), which is a closed-loop signal re-timing system.
We collaborated with a local traffic operation agency (Road Commissions for Oakland County) to perform a citywide field test of OSaaS in Birmingham, Michigan, with 34 signalized intersections. 2 corridors and 2 isolated intersections were diagnosed with improvement opportunity and implemented with new fixed-time signal timing plans, resulting in decreases in both the delay and number of stops by up to 20% and 30%, respectively. As shown by Figure 2, the coordination of one of the tested corridors was significantly improved after the offset optimization.